Daniel N. Bassett, Kurt Manal, Qi Shao, and Thomas S. Buchanan
Center for Biomedical Engineering Research, University of Delaware, Newark DE 19716
INTRODUCTION
Biomechanical models have been developed to analyze the motion of healthy individuals that walk with normal gait patterns. Such models are important for the study of muscle stimulation, prototype design, and limb control. We have created a biomechanical model of the ankle designed to predict joint moments in both unimpaired subjects and those who have had neuromuscular disorders. In this paper we will use this approach to study ankle moments in patients who have had strokes. Future research could apply the model to help subjects having abnormal gait patterns learn how to correct their muscle activation patterns through increased limb control and functional electrical stimulation.
METHODS
Three types of data were collected on normal and stroke affected subjects during isokinetic and gait trials: EMG from the tibialis anterior, medial gastrocnemius, lateral gastrocnemius, and soleus, joint position, and reaction forces (from the ground or dynamometer). Forward dynamics, using EMG and joint position data, was used to estimate the joint moments. This was verified by comparison with the inverse dynamics calculation.
The forward dynamics calculation was comprised of three elements: (1) muscle activation dynamics, (2) muscle contraction dynamics, and (3) musculoskeletal geometry. Muscle activation dynamics started from raw EMG which was rectified, filtered, and normalized. The EMG activation was then passed through a discretized recursive filter that gave neural activation. Muscle activation was calculated by non-linearizing neural activation. Muscle contraction dynamics was based on a Hill-type model approach deriving muscle force from a combination of active, passive, and fiber-velocity-dependent force which was calculated from muscle activation and the muscle tendon lengths [1]. Calculation of both activation and contraction dynamics involves the use of unknown physical parameters. Relevant musculoskeletal geometry was the muscle moment arms which, along with muscle force, gave total joint moment [2].
The model was calibrated by optimizing the forward dynamics joint moment to fit the inverse dynamics calculation. The calibration process was done by varying unknown parameters using simulated annealing [3]. Once the fit was achieved, the parameters were used in the forward dynamic prediction of joint moment for trials for which the model had not been calibrated.
RESULTS AND DISCUSSION
The results of the calibrations and predictions of joint moments for unimpaired and post-stroke subjects were very similar, (Figure 1). The joint moment patterns (from both the model and inverse dynamics), r-squared values, and RMS error were all comparable. Muscle forces and fiber lengths were consistent with literature, indicating the model is potentially a valuable tool to deliver realistic joint moment predictions.
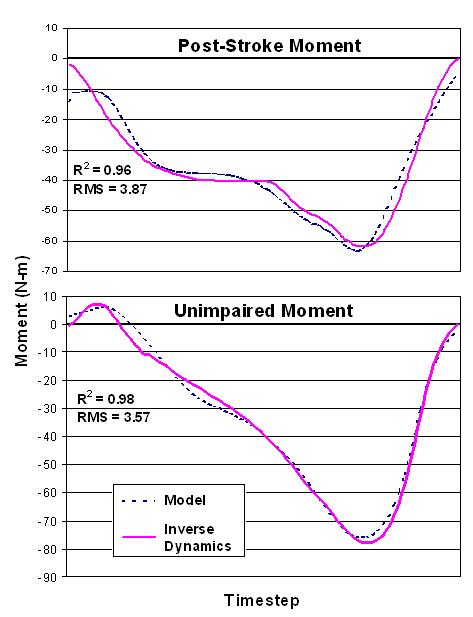
Figure 1: Comparison of calibration between a stroke patient and healthy subject
The differences noted between subject groups were in the muscle activations and force. According to the model, an unimpaired person produces the predominance of the joint moment with their medial gastrocnemius and soleus while walking, the rest created by the lateral gastrocnemius, implying insignificant torque contribution by the tibialis anterior. Whereas a post-stroke patient produces an antagonist moment with the tibialis anterior to compensate for the enlarged moment generated by the gastrocnemii and soleus. The discrepancy is not deemed to be an error of the model; it can be explained by the fact that an individual who has spasticity due to a stroke has increased triceps surae forces.
CONCLUSIONS
In our testing, the model was able to accurately predict joint moments in novel trials for subjects with normal and abnormal gait patterns.
REFERENCES
1. Delp SL, et al. Comput Biol Med 25(1), 21-34
2. Buchanan TS, et al. J App. Biomech, 2004, 20, 367-395.
3. Goffe WL, et al. J Econometrics, 1994, 60, 65-99
ACKNOWLEDGEMENTS
NIH R01-HD38582: FES and Biomechanics (TS Buchanan)
No comments:
Post a Comment