The American Society of Biomechanics conference was this last weekend, and it went very well. In spite of my laptop commiting technological suicide an hour before my talk, the presentation went very well. Herzog's talk as the recipient of the Borelli award was jaw dropping as he showed actual sarcomere's contracting. The close to the conference was inspiring as I attended the symposium on modelling which included great talks by Casey Carrigan, BJ Fregley, and Scott Delp.
In other news, a few very good possibilities for jobs have opened up in Italy. I have not received any formal offers as of yet, but will be interviewing hopefully in the next month.
Wednesday, September 13, 2006
Saturday, August 12, 2006
August 2006 News
My family and I are still in Newark, DE as I near finishing my Master's Degree at the University of Delaware. I recently met with my advisor, Dr. Buchanan, to discuss important dates, and we came up with the following:
-Proposal: September
-Defense: Early December
I am also actively seeking employment starting in January. I have found a few different options that I will be pursuing; hopefully, one of them will work out so we can continue to eat - I don't do well without my pasta!
Finally, I am preparing to present at the ASB meeting coming up in September. I should be able to get some better results than I presented at ASME to show the strength of my new multi-joint EMG driven model.
-Proposal: September
-Defense: Early December
I am also actively seeking employment starting in January. I have found a few different options that I will be pursuing; hopefully, one of them will work out so we can continue to eat - I don't do well without my pasta!
Finally, I am preparing to present at the ASB meeting coming up in September. I should be able to get some better results than I presented at ASME to show the strength of my new multi-joint EMG driven model.
Chapter XII in Begg & Palaniswami
Estimation of Muscle Forces About the Ankle During Gait in Healthy and Neurologically Impaired Subjects
Full Article (.pdf)
Daniel N. Bassett, Joseph D. Gardinier, Kurt T. Manal, Thomas S. Buchanan,
ABSTRACT
This chapter describes a biomechanical model of the forces about the ankle joint applicable to both unimpaired and neurologically impaired subjects. EMGs and joint kinematics are used as inputs and muscle forces are the outputs. A hybrid modeling approach that uses both forward and inverse dynamics is employed and physiological parameters for the model are tuned for each subject using optimization procedures. The forward dynamics part of the model takes muscle activation and uses Hill-type models of muscle contraction dynamics to estimate muscle forces and the corresponding joint moments. Inverse dynamics is used to calibrate the forward dynamics model predictions of joint moments. In this chapter we will describe how to implement an EMG-driven hybrid forward and inverse dynamics model of the ankle that can be used in healthy and neurologically impaired people.
Full Article (.pdf)
Daniel N. Bassett, Joseph D. Gardinier, Kurt T. Manal, Thomas S. Buchanan,
ABSTRACT
This chapter describes a biomechanical model of the forces about the ankle joint applicable to both unimpaired and neurologically impaired subjects. EMGs and joint kinematics are used as inputs and muscle forces are the outputs. A hybrid modeling approach that uses both forward and inverse dynamics is employed and physiological parameters for the model are tuned for each subject using optimization procedures. The forward dynamics part of the model takes muscle activation and uses Hill-type models of muscle contraction dynamics to estimate muscle forces and the corresponding joint moments. Inverse dynamics is used to calibrate the forward dynamics model predictions of joint moments. In this chapter we will describe how to implement an EMG-driven hybrid forward and inverse dynamics model of the ankle that can be used in healthy and neurologically impaired people.
ASB 2006
SINGLE JOINT VERSUS MULTIPLE JOINT MODELING USING A HYBRID-EMG DRIVEN APPROACH
Daniel N. Bassett1, Qi Shao1, Daniel L. Benoit1, Kurt T. Manal1, and Thomas S. Buchanan11 Center for Biomedical Engineering Research
University of Delaware, Newark, DE, USA E-mail: bassett@me.udel.edu Web: http://www.cber.udel.edu/
INTRODUCTION
When one is interested in modeling the forces in a joint it is tempting to focus only on that joint. The disadvantage of this approach is that it may not provide a sufficiently powerful representation of the way biarticular muscles contribute to single joints. In this study, our previous single joint model was expanded to include multiple joints. Specifically, we compared estimation of ankle joint moments and muscle forces with results from a combined ankle and knee model.
METHODS
The data collection for this preliminary comparison of modeling methods was conducted on a subject with healthy gait. The subject performed gait and maximum voluntary contraction trials. The data collected were kinematics, muscle specific electromyography (EMG), and ground reaction forces. Muscles chosen were the semitendinosus, biceps femoris, rectus femoris, vastus lateralis, vastus medialis about the knee (Lloyd & Besier, 2002), the tibialis anterior and soleus about the ankle, and the gastrocnemii as biarticular muscles that span both the ankle and knee.
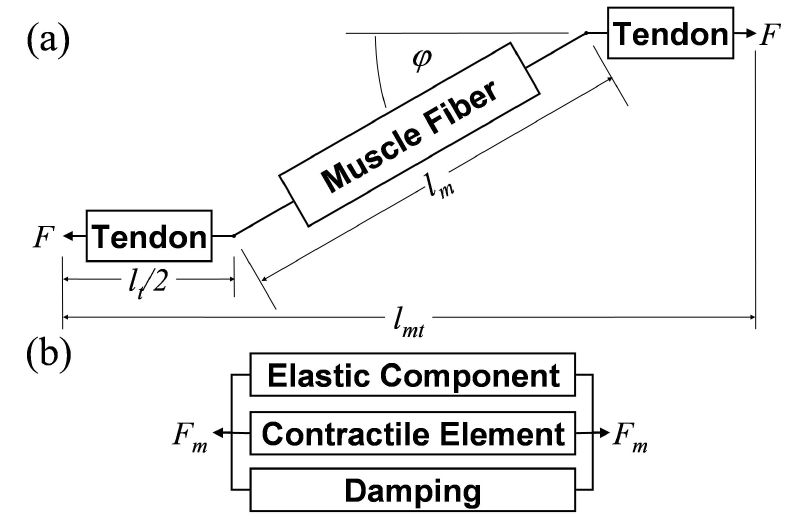
Figure 1: Hill-type model: a) muscle-tendon unit, b) muscle fiber unit. Where F is force, lt tendon length, lm muscle fiber length, lmt muscle-tendon length, φ pennation angle, and Fm muscle force.
After the data collection, we averaged the EMGs from the vasti estimating activation for the vastus intermedius. In addition, EMG for the biceps femoris was assumed to be the same for both the long and short head. EMG data were processed by relieving bias, rectifying, high and low-pass filtering, and finally normalizing by the maximal activation for each muscle. The kinematic data were used to obtain joint angles for the hip, knee, and ankle and subsequently muscle-tendon lengths and muscle moment arms using SIMM. Also, inverse dynamic joint moments were calculated for the knee and ankle from the kinematic data and the ground reaction forces.
Our EMG-driven model is built on a forward dynamic approach using a Hill-type model which includes active, passive, and damping components (Figure 1) (Buchanan et al., 2005). The processed EMG data were passed through a history-dependent recursive filter and then non-linearized to give muscle activation. The equation relating components of our Hill-type model was integrated to calculate fiber length and tendon length. Tendon force was interpolated from the force-length relationship, which combined with muscle moment arms gave joint moments (Buchanan et al., 2004).
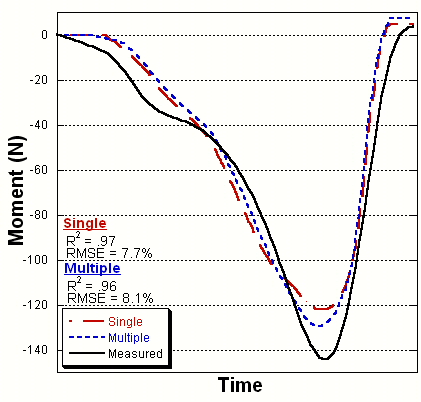
Figure 2: Ankle joint moment comparison
Due to the difficulty of in vivo measurement of subject specific muscle parameters, such as tendon slack length, we used a hybrid model. In the tuning process, these parameters were adjusted according to an optimization algorithm (Goffe et al., 1994) using the inverse dynamic joint moments as the standard. Our model was tuned to the ankle and then to the ankle and knee combined for the first trial. The tuned models were then used to predict ankle joint moments for other walking trials.
RESULTS AND DISCUSSION
The model’s ability to predict joint moments was consistent between single and multiple joint calibrations (R2 = 0.97 and 0.96 respectively). RMS values of 7.7% and 8.1% showed a moderate increase in error when the knee was included in the tuning process (Figure 2). The RMS difference between the two predictions was 1.3%, and a 5% reduction in peak error was found by using the multi-joint model. The differences found between our models are seen in the muscle forces. The predictions for the soleus and tibialis anterior varied less than 5% between the two calibrations. On the other hand, the forces were estimated to change up to 20 percent for the gastrocnemii in the combined model.
The study showed consistency in joint moment predictions. More importantly, the deviations in muscle force predictions were more pronounced for the biarticular muscles, as expected. Single joint modeling of the ankle neglects contributions of the gastrocnemii at the knee. Multiple joint modeling accounts for more complex and physiological kinetics.
CONCLUSIONS
The performance of our multiple joint model was consistent with our previous work. The differences found in muscle force estimation are most likely due to increased physiological accuracy of the model.
REFERENCES
Buchanan, T.S., Lloyd, D.G., Manal, K.T., Besier, T.F. (2004). J. App. Biomech, 20, 367-395.
Buchanan, T.S., Lloyd, D.G., Manal, K.T., Besier, T.F. (2005). Med Sci Sports Exerc., 1911-1916.
Goffe, W.L., Ferrier, G.D., Rogers, J. (1994). J. Econom., 60, 65-99.
Lloyd, D.G., Besier, T.F. (2002). J. Biomech., 36, 765-776.
ACKNOWLEDGEMENTS
NIH R01-HD38582 and P20-RR16458
Daniel N. Bassett1, Qi Shao1, Daniel L. Benoit1, Kurt T. Manal1, and Thomas S. Buchanan11 Center for Biomedical Engineering Research
University of Delaware, Newark, DE, USA E-mail: bassett@me.udel.edu Web: http://www.cber.udel.edu/
INTRODUCTION
When one is interested in modeling the forces in a joint it is tempting to focus only on that joint. The disadvantage of this approach is that it may not provide a sufficiently powerful representation of the way biarticular muscles contribute to single joints. In this study, our previous single joint model was expanded to include multiple joints. Specifically, we compared estimation of ankle joint moments and muscle forces with results from a combined ankle and knee model.
METHODS
The data collection for this preliminary comparison of modeling methods was conducted on a subject with healthy gait. The subject performed gait and maximum voluntary contraction trials. The data collected were kinematics, muscle specific electromyography (EMG), and ground reaction forces. Muscles chosen were the semitendinosus, biceps femoris, rectus femoris, vastus lateralis, vastus medialis about the knee (Lloyd & Besier, 2002), the tibialis anterior and soleus about the ankle, and the gastrocnemii as biarticular muscles that span both the ankle and knee.
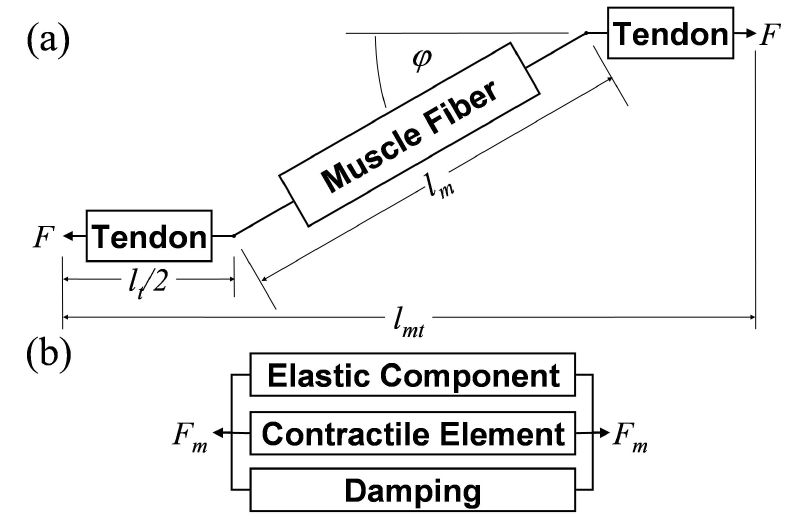
Figure 1: Hill-type model: a) muscle-tendon unit, b) muscle fiber unit. Where F is force, lt tendon length, lm muscle fiber length, lmt muscle-tendon length, φ pennation angle, and Fm muscle force.
After the data collection, we averaged the EMGs from the vasti estimating activation for the vastus intermedius. In addition, EMG for the biceps femoris was assumed to be the same for both the long and short head. EMG data were processed by relieving bias, rectifying, high and low-pass filtering, and finally normalizing by the maximal activation for each muscle. The kinematic data were used to obtain joint angles for the hip, knee, and ankle and subsequently muscle-tendon lengths and muscle moment arms using SIMM. Also, inverse dynamic joint moments were calculated for the knee and ankle from the kinematic data and the ground reaction forces.
Our EMG-driven model is built on a forward dynamic approach using a Hill-type model which includes active, passive, and damping components (Figure 1) (Buchanan et al., 2005). The processed EMG data were passed through a history-dependent recursive filter and then non-linearized to give muscle activation. The equation relating components of our Hill-type model was integrated to calculate fiber length and tendon length. Tendon force was interpolated from the force-length relationship, which combined with muscle moment arms gave joint moments (Buchanan et al., 2004).
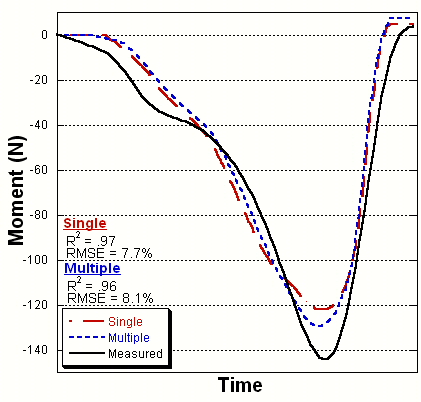
Figure 2: Ankle joint moment comparison
Due to the difficulty of in vivo measurement of subject specific muscle parameters, such as tendon slack length, we used a hybrid model. In the tuning process, these parameters were adjusted according to an optimization algorithm (Goffe et al., 1994) using the inverse dynamic joint moments as the standard. Our model was tuned to the ankle and then to the ankle and knee combined for the first trial. The tuned models were then used to predict ankle joint moments for other walking trials.
RESULTS AND DISCUSSION
The model’s ability to predict joint moments was consistent between single and multiple joint calibrations (R2 = 0.97 and 0.96 respectively). RMS values of 7.7% and 8.1% showed a moderate increase in error when the knee was included in the tuning process (Figure 2). The RMS difference between the two predictions was 1.3%, and a 5% reduction in peak error was found by using the multi-joint model. The differences found between our models are seen in the muscle forces. The predictions for the soleus and tibialis anterior varied less than 5% between the two calibrations. On the other hand, the forces were estimated to change up to 20 percent for the gastrocnemii in the combined model.
The study showed consistency in joint moment predictions. More importantly, the deviations in muscle force predictions were more pronounced for the biarticular muscles, as expected. Single joint modeling of the ankle neglects contributions of the gastrocnemii at the knee. Multiple joint modeling accounts for more complex and physiological kinetics.
CONCLUSIONS
The performance of our multiple joint model was consistent with our previous work. The differences found in muscle force estimation are most likely due to increased physiological accuracy of the model.
REFERENCES
Buchanan, T.S., Lloyd, D.G., Manal, K.T., Besier, T.F. (2004). J. App. Biomech, 20, 367-395.
Buchanan, T.S., Lloyd, D.G., Manal, K.T., Besier, T.F. (2005). Med Sci Sports Exerc., 1911-1916.
Goffe, W.L., Ferrier, G.D., Rogers, J. (1994). J. Econom., 60, 65-99.
Lloyd, D.G., Besier, T.F. (2002). J. Biomech., 36, 765-776.
ACKNOWLEDGEMENTS
NIH R01-HD38582 and P20-RR16458
ASME Summer Bioengineering 2006
PREDICTING ANKLE AND KNEE JOINT MOMENTS USING A HYBRID-EMG DRIVEN MODEL ON EACH JOINT INDIVIDUALLY AND COMBINED
Daniel N. Bassett, Qi Shao, Daniel L Benoit, Kurt T. Manal, Thomas S. Buchanan
Center for Biomedical Engineering Research
University of Delaware
Newark, DE
INTRODUCTION
The implementation of biomechanical EMG-driven models has become more widespread in recent years. One important application for such modeling is in the analysis of muscle forces during gait. Such models generally focus on a single joint (i.e., the knee or ankle) due to the problems of collecting EMGs from so many muscles. However, although the models must balance the forces for any particular joint, models that take into account multiple joints simultaneously may yield different solutions due to the way biarticular muscles are reckoned.
In this study, we present an expanded version of our model that allows us to account for multiple joints. We used this model to predict joint moments for the ankle and the knee in two ways: treating each of the joints separately and treating them both together. We then compared the results obtained using these two methods to evaluate the differences in the prediction of joint moments and muscles forces.
METHODS
Data Collection
For this preliminary study, we collected data from one healthy individual possessing a normal gait pattern and moderate physically active background. The subject performed multiple maximum voluntary contractions (MVC) and gait trials. Kinematic data were recorded using a Qualysis motion system. Ground reaction forces were obtained by means of an in-floor force plate, and activity from nine muscles was recorded using electromyography (EMG). The muscles about the knee were chosen in accordance with Lloyd [1] and included the semitendinosus, biceps femoris, rectus femoris, vastus lateralis, vastus medialis, gastrocnemius lateralis, and gastrocnemius medialis. For muscles about the ankle, EMGs were collected from the soleus (Sol) and tibialis anterior (TA) beyond the medial and lateral gastrocnemii (GM and GL) already included at the knee.
Data Processing
In addition to the nine muscles we collected from, we averaged the EMG for the medial and lateral vasti to obtain data for the vastus intermedius. Also, the biceps femoris was assumed to have the same EMG for the long head and short head.
The EMG was relieved of bias, then rectified, high-pass filtered, low-pass filtered, and normalized by the maximal activation from the MVC trials, giving us vales we termed “EMG activation” [2].
The kinematic data were used to calculate joint angles for the hip, knee, and ankle, from which muscle tendon lengths and muscle moment arms were determined using SIMM for each of the eleven muscles analyzed. Furthermore, the kinematic data in combination with the ground reaction forces were used to estimate joint moments by inverse dynamics.
Biomechanical Model
The model we use is forward dynamic and EMG-driven based on a Hill-type model which includes active, passive, and damping components [3].
(1)
Equation (1) displays the relationship between the three components, and includes muscle force (F), max isometric force (Fmax), active force (FA), velocity dependent force (FV), passive force (FP), muscle activation (a(t)), muscle fiber length (lm), muscle fiber velocity (vm), damping (bm), and pennation angle (φ).
Muscle activation was obtained by passing EMG activation through a history dependent recursive filter and then non-linearizing it. Muscle fiber length was then calculated by using a forward integration based on equation (1), and recalling that the force passing through the tendon must be equal to that produced by the muscle. Once the muscle fiber length is known it was subtracted from the muscle tendon length giving the length of the tendon. Tendon force was interpolated using the corresponding force-length relationship. Finally, joint moments can be easily obtained from muscle forces and muscle moment arms.
Subject specific muscle parameters such as tendon slack length are difficult to measure accurately in vivo. For this reason we converted our forward dynamic model into a hybrid model during the tuning process and tuned these parameters for each subject (within physiological bounds). Model parameters were adjusted using an optimization algorithm [4] so that the joint moments calculated from inverse dynamics matched those of the forward dynamic calculations. Model tuning was performed three different times on the first trial: ankle, knee, and ankle and knee combined. The calibrated models were then used for joint moment predictions on novel data.
results &Discussion
Analysis of the joint moments produced by applying the three different calibrations revealed consistency in the model’s predictions. Tuning the model with two joints simultaneously as opposed to one increased the error a modest amount. In Figure 1, it can be seen that the joint moment patterns are closely correlated. The R2 values were 0.97 for the individual optimization and 0.96 for the combined calibration; the RMS errors were 7.7% and 8.1% respectively. The difference between the two predictions was 1.3% (RMS) for the ankle.
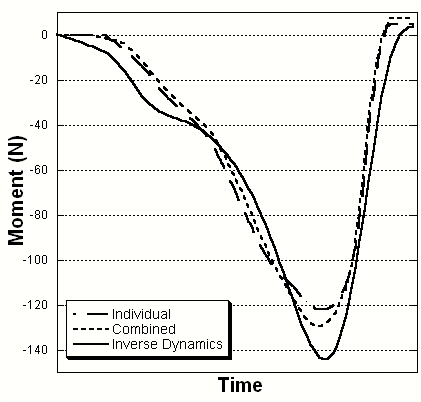
Figure 1. Ankle Joint Moment Comparison
The differences in estimated forces for muscles around the ankle are shown in Figure 2. The different optimizations resulted in little deviation in muscle force estimation between the soleus and tibialis anterior. However, the gastrocnemii force predictions were on average between 15 and 20 percent different between calibrations. The RMS deviations showed a decrease in force from individual to combined tuning of 170 N for the GM and 13 N for the GL.
This study showed that our model was able to estimate joint moments when accounting for multiple joints with similar accuracy as
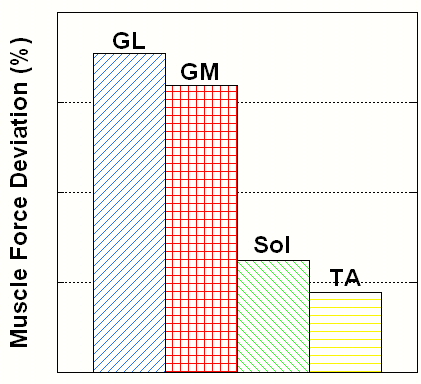
Figure 2. Muscle Force Deviation between Ankle and Combined Ankle and Knee Optimizations
when only examining a single joint. More importantly, however, is the model’s ability to predict muscle forces. The force deviations between ankle joint and combined calibration were more evident in the GM and GL compared to the Sol and TA. This was expected since the gastrocnemii are the only biarticular muscles spanning both ankle and knee. When optimizing parameters for muscles about the ankle exclusively, the contributions to the knee are neglected. On the other hand, when including the knee, the results for the gastrocnemii are going to be dependent on more complex kinetics and consequently more realistic. Verification of the results should provide additional insight into the differences in the forces estimates. We are developing a way to do this by comparing model estimated tendon strain with measures determined from ultrasound.
Conclusion
In this study, the multi-joint model made reasonable predictions of joint moments, with errors close to those of single joint models. Although there are differences associated with the estimated muscle forces when comparing the two models, we believe the multi-joint model provides more physiologically accuracy.
References
1. Lloyd, D.G., & Besier, T.F., 2002, “An EMG-driven musculoskeletal model to estimate muscle forces and knee joint moments in vivo,” Journal of Biomechanics, 36, pp. 765-776.
2. Buchanan, T.S., Lloyd, D.G., Manal, K.T., & Besier, T.F., 2005, “Estimation of muscle forces and joint moments using a forward-inverse dynamics model,” Medicine & Science in Sports & Exercise, pp. 1911-1916.
3. Buchanan, T.S., Lloyd, D.G., Manal, K.T., & Besier, T.F., 2004, “Neuromusculoskeletal modeling: estimation of muscle forces and joint moments and movements from measurements of neural command,” Journal of Applied Biomechanics, 20, pp. 367-395.
4. Goffe, W.L., Ferrier, G.D., & Rogers, J., 1994, “Global optimization of statistical functions with simulated annealing. Journal of Econometrics,” 60, pp. 65-99.
Acknowledgements
NIH R01-HD38582 and P20-RR16458.
Daniel N. Bassett, Qi Shao, Daniel L Benoit, Kurt T. Manal, Thomas S. Buchanan
Center for Biomedical Engineering Research
University of Delaware
Newark, DE
INTRODUCTION
The implementation of biomechanical EMG-driven models has become more widespread in recent years. One important application for such modeling is in the analysis of muscle forces during gait. Such models generally focus on a single joint (i.e., the knee or ankle) due to the problems of collecting EMGs from so many muscles. However, although the models must balance the forces for any particular joint, models that take into account multiple joints simultaneously may yield different solutions due to the way biarticular muscles are reckoned.
In this study, we present an expanded version of our model that allows us to account for multiple joints. We used this model to predict joint moments for the ankle and the knee in two ways: treating each of the joints separately and treating them both together. We then compared the results obtained using these two methods to evaluate the differences in the prediction of joint moments and muscles forces.
METHODS
Data Collection
For this preliminary study, we collected data from one healthy individual possessing a normal gait pattern and moderate physically active background. The subject performed multiple maximum voluntary contractions (MVC) and gait trials. Kinematic data were recorded using a Qualysis motion system. Ground reaction forces were obtained by means of an in-floor force plate, and activity from nine muscles was recorded using electromyography (EMG). The muscles about the knee were chosen in accordance with Lloyd [1] and included the semitendinosus, biceps femoris, rectus femoris, vastus lateralis, vastus medialis, gastrocnemius lateralis, and gastrocnemius medialis. For muscles about the ankle, EMGs were collected from the soleus (Sol) and tibialis anterior (TA) beyond the medial and lateral gastrocnemii (GM and GL) already included at the knee.
Data Processing
In addition to the nine muscles we collected from, we averaged the EMG for the medial and lateral vasti to obtain data for the vastus intermedius. Also, the biceps femoris was assumed to have the same EMG for the long head and short head.
The EMG was relieved of bias, then rectified, high-pass filtered, low-pass filtered, and normalized by the maximal activation from the MVC trials, giving us vales we termed “EMG activation” [2].
The kinematic data were used to calculate joint angles for the hip, knee, and ankle, from which muscle tendon lengths and muscle moment arms were determined using SIMM for each of the eleven muscles analyzed. Furthermore, the kinematic data in combination with the ground reaction forces were used to estimate joint moments by inverse dynamics.
Biomechanical Model
The model we use is forward dynamic and EMG-driven based on a Hill-type model which includes active, passive, and damping components [3].

Equation (1) displays the relationship between the three components, and includes muscle force (F), max isometric force (Fmax), active force (FA), velocity dependent force (FV), passive force (FP), muscle activation (a(t)), muscle fiber length (lm), muscle fiber velocity (vm), damping (bm), and pennation angle (φ).
Muscle activation was obtained by passing EMG activation through a history dependent recursive filter and then non-linearizing it. Muscle fiber length was then calculated by using a forward integration based on equation (1), and recalling that the force passing through the tendon must be equal to that produced by the muscle. Once the muscle fiber length is known it was subtracted from the muscle tendon length giving the length of the tendon. Tendon force was interpolated using the corresponding force-length relationship. Finally, joint moments can be easily obtained from muscle forces and muscle moment arms.
Subject specific muscle parameters such as tendon slack length are difficult to measure accurately in vivo. For this reason we converted our forward dynamic model into a hybrid model during the tuning process and tuned these parameters for each subject (within physiological bounds). Model parameters were adjusted using an optimization algorithm [4] so that the joint moments calculated from inverse dynamics matched those of the forward dynamic calculations. Model tuning was performed three different times on the first trial: ankle, knee, and ankle and knee combined. The calibrated models were then used for joint moment predictions on novel data.
results &Discussion
Analysis of the joint moments produced by applying the three different calibrations revealed consistency in the model’s predictions. Tuning the model with two joints simultaneously as opposed to one increased the error a modest amount. In Figure 1, it can be seen that the joint moment patterns are closely correlated. The R2 values were 0.97 for the individual optimization and 0.96 for the combined calibration; the RMS errors were 7.7% and 8.1% respectively. The difference between the two predictions was 1.3% (RMS) for the ankle.
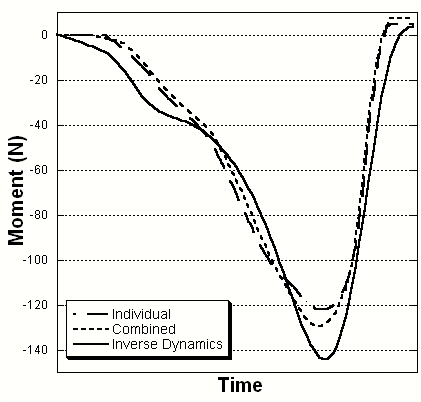
Figure 1. Ankle Joint Moment Comparison
The differences in estimated forces for muscles around the ankle are shown in Figure 2. The different optimizations resulted in little deviation in muscle force estimation between the soleus and tibialis anterior. However, the gastrocnemii force predictions were on average between 15 and 20 percent different between calibrations. The RMS deviations showed a decrease in force from individual to combined tuning of 170 N for the GM and 13 N for the GL.
This study showed that our model was able to estimate joint moments when accounting for multiple joints with similar accuracy as
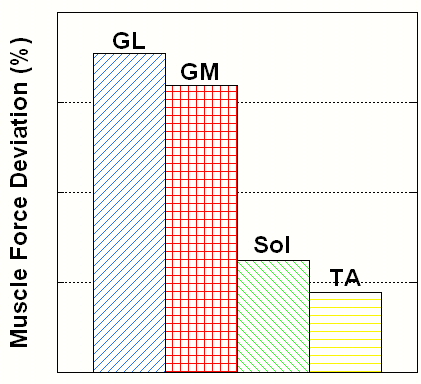
Figure 2. Muscle Force Deviation between Ankle and Combined Ankle and Knee Optimizations
when only examining a single joint. More importantly, however, is the model’s ability to predict muscle forces. The force deviations between ankle joint and combined calibration were more evident in the GM and GL compared to the Sol and TA. This was expected since the gastrocnemii are the only biarticular muscles spanning both ankle and knee. When optimizing parameters for muscles about the ankle exclusively, the contributions to the knee are neglected. On the other hand, when including the knee, the results for the gastrocnemii are going to be dependent on more complex kinetics and consequently more realistic. Verification of the results should provide additional insight into the differences in the forces estimates. We are developing a way to do this by comparing model estimated tendon strain with measures determined from ultrasound.
Conclusion
In this study, the multi-joint model made reasonable predictions of joint moments, with errors close to those of single joint models. Although there are differences associated with the estimated muscle forces when comparing the two models, we believe the multi-joint model provides more physiologically accuracy.
References
1. Lloyd, D.G., & Besier, T.F., 2002, “An EMG-driven musculoskeletal model to estimate muscle forces and knee joint moments in vivo,” Journal of Biomechanics, 36, pp. 765-776.
2. Buchanan, T.S., Lloyd, D.G., Manal, K.T., & Besier, T.F., 2005, “Estimation of muscle forces and joint moments using a forward-inverse dynamics model,” Medicine & Science in Sports & Exercise, pp. 1911-1916.
3. Buchanan, T.S., Lloyd, D.G., Manal, K.T., & Besier, T.F., 2004, “Neuromusculoskeletal modeling: estimation of muscle forces and joint moments and movements from measurements of neural command,” Journal of Applied Biomechanics, 20, pp. 367-395.
4. Goffe, W.L., Ferrier, G.D., & Rogers, J., 1994, “Global optimization of statistical functions with simulated annealing. Journal of Econometrics,” 60, pp. 65-99.
Acknowledgements
NIH R01-HD38582 and P20-RR16458.
CBER Day 2006
KNEE JOINT MOMENT CONTRIBUTION TO ANKLE JOINT MOMENT PREDICTION USING AN EMG-DRIVEN MODEL
Daniel N. Bassett, Qi Shao, Daniel L. Benoit, Kurt T. Manal, Thomas S. Buchanan
Center for Biomedical Engineering Research, University of Delaware, Newark, DE
INTRODUCTION: EMG-driven models are typically limited to one joint without accounting for moment contributions of biarticular muscles to other joints. The purpose of this study was to investigate the effect of accounting for moment contributions of biarticular ankle joint muscles in predicting ankle joint moments by comparing the ankle and multi-joint ankle/knee model predictions.
METHODS: EMGs were recorded from eleven muscles about the ankle and knee during gait in healthy subjects. Kinematic data were used to estimate muscle tendon lengths and moment arms, and then combined with ground reaction forces to calculate inverse dynamic joint moments for the ankle and knee. Our EMG-driven Hill-type model (Buchanan et al., 2005) was used to estimate joint moments based on a forward dynamics approach. The muscle model requires parameters that are hard to measure in vivo and must therefore be optimized (Goffe at al 1994) using the inverse dynamic joint moments as the criterion. We calibrated both the ankle and multi-joint models to predict joint moments for novel trials.
RESULTS & DISCUSSION: The joint moments predicted by the models were compared to the inverse dynamic joint moments, and found to be correlated and accurate for both ankle (R2=0.97; RMS=7.7%) and multi-joint (R2=0.96; RMS=8.1%) predictions. However, muscle forces of the biarticular gastrocnemii changed by 15-20% when the knee was included in the model. The differences found in muscle force estimation are most likely due to improved physiological accuracy of the model.
REFERENCES: [1] Buchanan TS, et al., Estimation of muscle forces and joint moments using a forward-inverse dynamics model, Med Sci Sports Exerc. 37:1911-1916, 2005. [2] Goffe WL, et al., Global optimization of statistical functions with simulated annealing. J Econometrics., 60:65-99,1994
Daniel N. Bassett, Qi Shao, Daniel L. Benoit, Kurt T. Manal, Thomas S. Buchanan
Center for Biomedical Engineering Research, University of Delaware, Newark, DE
INTRODUCTION: EMG-driven models are typically limited to one joint without accounting for moment contributions of biarticular muscles to other joints. The purpose of this study was to investigate the effect of accounting for moment contributions of biarticular ankle joint muscles in predicting ankle joint moments by comparing the ankle and multi-joint ankle/knee model predictions.
METHODS: EMGs were recorded from eleven muscles about the ankle and knee during gait in healthy subjects. Kinematic data were used to estimate muscle tendon lengths and moment arms, and then combined with ground reaction forces to calculate inverse dynamic joint moments for the ankle and knee. Our EMG-driven Hill-type model (Buchanan et al., 2005) was used to estimate joint moments based on a forward dynamics approach. The muscle model requires parameters that are hard to measure in vivo and must therefore be optimized (Goffe at al 1994) using the inverse dynamic joint moments as the criterion. We calibrated both the ankle and multi-joint models to predict joint moments for novel trials.
RESULTS & DISCUSSION: The joint moments predicted by the models were compared to the inverse dynamic joint moments, and found to be correlated and accurate for both ankle (R2=0.97; RMS=7.7%) and multi-joint (R2=0.96; RMS=8.1%) predictions. However, muscle forces of the biarticular gastrocnemii changed by 15-20% when the knee was included in the model. The differences found in muscle force estimation are most likely due to improved physiological accuracy of the model.
REFERENCES: [1] Buchanan TS, et al., Estimation of muscle forces and joint moments using a forward-inverse dynamics model, Med Sci Sports Exerc. 37:1911-1916, 2005. [2] Goffe WL, et al., Global optimization of statistical functions with simulated annealing. J Econometrics., 60:65-99,1994
ISB 2005
PREDICTING ANKLE JOINT MOMENT IN SUBJECTS WITH NORMAL OR ABNORMAL GAIT PATTERN
Daniel N. Bassett, Kurt Manal, Qi Shao, and Thomas S. Buchanan
Center for Biomedical Engineering Research, University of Delaware, Newark DE 19716
INTRODUCTION
Biomechanical models have been developed to analyze the motion of healthy individuals that walk with normal gait patterns. Such models are important for the study of muscle stimulation, prototype design, and limb control. We have created a biomechanical model of the ankle designed to predict joint moments in both unimpaired subjects and those who have had neuromuscular disorders. In this paper we will use this approach to study ankle moments in patients who have had strokes. Future research could apply the model to help subjects having abnormal gait patterns learn how to correct their muscle activation patterns through increased limb control and functional electrical stimulation.
METHODS
Three types of data were collected on normal and stroke affected subjects during isokinetic and gait trials: EMG from the tibialis anterior, medial gastrocnemius, lateral gastrocnemius, and soleus, joint position, and reaction forces (from the ground or dynamometer). Forward dynamics, using EMG and joint position data, was used to estimate the joint moments. This was verified by comparison with the inverse dynamics calculation.
The forward dynamics calculation was comprised of three elements: (1) muscle activation dynamics, (2) muscle contraction dynamics, and (3) musculoskeletal geometry. Muscle activation dynamics started from raw EMG which was rectified, filtered, and normalized. The EMG activation was then passed through a discretized recursive filter that gave neural activation. Muscle activation was calculated by non-linearizing neural activation. Muscle contraction dynamics was based on a Hill-type model approach deriving muscle force from a combination of active, passive, and fiber-velocity-dependent force which was calculated from muscle activation and the muscle tendon lengths [1]. Calculation of both activation and contraction dynamics involves the use of unknown physical parameters. Relevant musculoskeletal geometry was the muscle moment arms which, along with muscle force, gave total joint moment [2].
The model was calibrated by optimizing the forward dynamics joint moment to fit the inverse dynamics calculation. The calibration process was done by varying unknown parameters using simulated annealing [3]. Once the fit was achieved, the parameters were used in the forward dynamic prediction of joint moment for trials for which the model had not been calibrated.
RESULTS AND DISCUSSION
The results of the calibrations and predictions of joint moments for unimpaired and post-stroke subjects were very similar, (Figure 1). The joint moment patterns (from both the model and inverse dynamics), r-squared values, and RMS error were all comparable. Muscle forces and fiber lengths were consistent with literature, indicating the model is potentially a valuable tool to deliver realistic joint moment predictions.
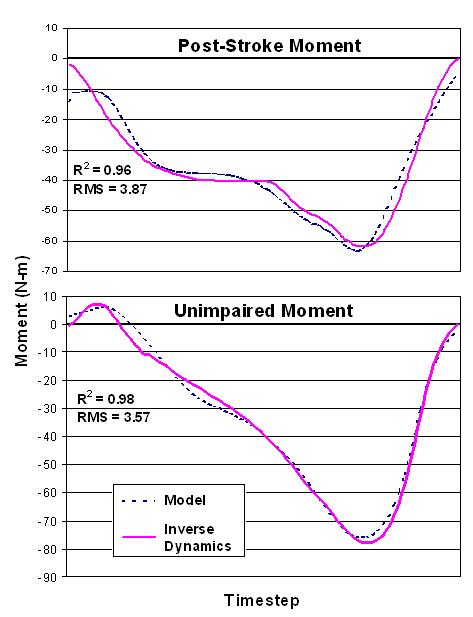
Figure 1: Comparison of calibration between a stroke patient and healthy subject
The differences noted between subject groups were in the muscle activations and force. According to the model, an unimpaired person produces the predominance of the joint moment with their medial gastrocnemius and soleus while walking, the rest created by the lateral gastrocnemius, implying insignificant torque contribution by the tibialis anterior. Whereas a post-stroke patient produces an antagonist moment with the tibialis anterior to compensate for the enlarged moment generated by the gastrocnemii and soleus. The discrepancy is not deemed to be an error of the model; it can be explained by the fact that an individual who has spasticity due to a stroke has increased triceps surae forces.
CONCLUSIONS
In our testing, the model was able to accurately predict joint moments in novel trials for subjects with normal and abnormal gait patterns.
REFERENCES
1. Delp SL, et al. Comput Biol Med 25(1), 21-34
2. Buchanan TS, et al. J App. Biomech, 2004, 20, 367-395.
3. Goffe WL, et al. J Econometrics, 1994, 60, 65-99
ACKNOWLEDGEMENTS
NIH R01-HD38582: FES and Biomechanics (TS Buchanan)
Daniel N. Bassett, Kurt Manal, Qi Shao, and Thomas S. Buchanan
Center for Biomedical Engineering Research, University of Delaware, Newark DE 19716
INTRODUCTION
Biomechanical models have been developed to analyze the motion of healthy individuals that walk with normal gait patterns. Such models are important for the study of muscle stimulation, prototype design, and limb control. We have created a biomechanical model of the ankle designed to predict joint moments in both unimpaired subjects and those who have had neuromuscular disorders. In this paper we will use this approach to study ankle moments in patients who have had strokes. Future research could apply the model to help subjects having abnormal gait patterns learn how to correct their muscle activation patterns through increased limb control and functional electrical stimulation.
METHODS
Three types of data were collected on normal and stroke affected subjects during isokinetic and gait trials: EMG from the tibialis anterior, medial gastrocnemius, lateral gastrocnemius, and soleus, joint position, and reaction forces (from the ground or dynamometer). Forward dynamics, using EMG and joint position data, was used to estimate the joint moments. This was verified by comparison with the inverse dynamics calculation.
The forward dynamics calculation was comprised of three elements: (1) muscle activation dynamics, (2) muscle contraction dynamics, and (3) musculoskeletal geometry. Muscle activation dynamics started from raw EMG which was rectified, filtered, and normalized. The EMG activation was then passed through a discretized recursive filter that gave neural activation. Muscle activation was calculated by non-linearizing neural activation. Muscle contraction dynamics was based on a Hill-type model approach deriving muscle force from a combination of active, passive, and fiber-velocity-dependent force which was calculated from muscle activation and the muscle tendon lengths [1]. Calculation of both activation and contraction dynamics involves the use of unknown physical parameters. Relevant musculoskeletal geometry was the muscle moment arms which, along with muscle force, gave total joint moment [2].
The model was calibrated by optimizing the forward dynamics joint moment to fit the inverse dynamics calculation. The calibration process was done by varying unknown parameters using simulated annealing [3]. Once the fit was achieved, the parameters were used in the forward dynamic prediction of joint moment for trials for which the model had not been calibrated.
RESULTS AND DISCUSSION
The results of the calibrations and predictions of joint moments for unimpaired and post-stroke subjects were very similar, (Figure 1). The joint moment patterns (from both the model and inverse dynamics), r-squared values, and RMS error were all comparable. Muscle forces and fiber lengths were consistent with literature, indicating the model is potentially a valuable tool to deliver realistic joint moment predictions.
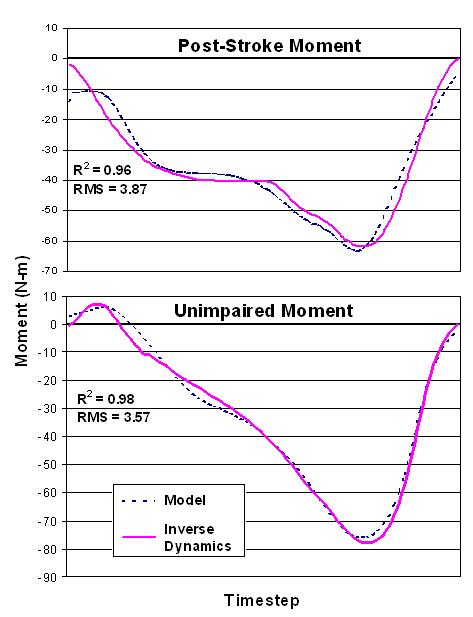
Figure 1: Comparison of calibration between a stroke patient and healthy subject
The differences noted between subject groups were in the muscle activations and force. According to the model, an unimpaired person produces the predominance of the joint moment with their medial gastrocnemius and soleus while walking, the rest created by the lateral gastrocnemius, implying insignificant torque contribution by the tibialis anterior. Whereas a post-stroke patient produces an antagonist moment with the tibialis anterior to compensate for the enlarged moment generated by the gastrocnemii and soleus. The discrepancy is not deemed to be an error of the model; it can be explained by the fact that an individual who has spasticity due to a stroke has increased triceps surae forces.
CONCLUSIONS
In our testing, the model was able to accurately predict joint moments in novel trials for subjects with normal and abnormal gait patterns.
REFERENCES
1. Delp SL, et al. Comput Biol Med 25(1), 21-34
2. Buchanan TS, et al. J App. Biomech, 2004, 20, 367-395.
3. Goffe WL, et al. J Econometrics, 1994, 60, 65-99
ACKNOWLEDGEMENTS
NIH R01-HD38582: FES and Biomechanics (TS Buchanan)
Friday, August 11, 2006
ACSM 2005
Predicting Ankle Joint Moments using a Hybrid EMG-driven Model
Daniel N. Bassett, Kurt Manal, Shay Cohen, Thomas S. Buchanan.
University of Delaware, Newark, DE.
INTRODUCTION: To study sport medicine injuries and their effects on human motion, accurate biomechanical models are important. For these models to characterize the response to injury, they must take into account the differences in the ways injured people move their limbs and activate their muscles. We have created a biomechanical model of the ankle that uses subject specific muscle activation as an input and predicts the corresponding joint moment (plantar-flexion/dorsiflexion).
PURPOSE: To demonstrate the ability of our model to accurately predict joint moments given electromyograms (EMGs) and joint position data.
METHODS: Three types of data were collected during isokinetic and gait trials: (1) EMG from the tibialis anterior, medial gastrocnemius, lateral gastrocnemius, and soleus; (2) joint position, (3) and reaction forces (from the ground or dynamometer). Ankle joint moment was calculated in two ways: (1) forward dynamics using EMG and joint position data, and (2) inverse dynamics using joint position and reaction force data. The joint moment determined from the inverse dynamics calculation was used to calibrate the forward dynamic estimation of moment. Adjustable parameters in the forwards dynamics model were optimized to produce a best fit. Once calibrated, only the forward dynamics model was used to predict joint moment from novel trials.
RESULTS: Preliminary data were collected from three test subjects. A comparison between the predicted and the measured ankle joint moment was performed; root mean squared error was approximately 10%, and R-squared values were of 0.95, 0.95, 0.87 for each subject respectively.
CONCLUSIONS: The curve shape was very closely matched in the prediction of concentric tasks, but the largest errors were observed during eccentric tasks. Further adjustment and refinement of the model parameters should correct this. One of the major strengths of the model is that is allows estimation of muscle forces (in contrast to inverse dynamics models) and relies on a subject’s actual muscle activation values (in contrast to optimization approaches).
Daniel N. Bassett, Kurt Manal, Shay Cohen, Thomas S. Buchanan.
University of Delaware, Newark, DE.
INTRODUCTION: To study sport medicine injuries and their effects on human motion, accurate biomechanical models are important. For these models to characterize the response to injury, they must take into account the differences in the ways injured people move their limbs and activate their muscles. We have created a biomechanical model of the ankle that uses subject specific muscle activation as an input and predicts the corresponding joint moment (plantar-flexion/dorsiflexion).
PURPOSE: To demonstrate the ability of our model to accurately predict joint moments given electromyograms (EMGs) and joint position data.
METHODS: Three types of data were collected during isokinetic and gait trials: (1) EMG from the tibialis anterior, medial gastrocnemius, lateral gastrocnemius, and soleus; (2) joint position, (3) and reaction forces (from the ground or dynamometer). Ankle joint moment was calculated in two ways: (1) forward dynamics using EMG and joint position data, and (2) inverse dynamics using joint position and reaction force data. The joint moment determined from the inverse dynamics calculation was used to calibrate the forward dynamic estimation of moment. Adjustable parameters in the forwards dynamics model were optimized to produce a best fit. Once calibrated, only the forward dynamics model was used to predict joint moment from novel trials.
RESULTS: Preliminary data were collected from three test subjects. A comparison between the predicted and the measured ankle joint moment was performed; root mean squared error was approximately 10%, and R-squared values were of 0.95, 0.95, 0.87 for each subject respectively.
CONCLUSIONS: The curve shape was very closely matched in the prediction of concentric tasks, but the largest errors were observed during eccentric tasks. Further adjustment and refinement of the model parameters should correct this. One of the major strengths of the model is that is allows estimation of muscle forces (in contrast to inverse dynamics models) and relies on a subject’s actual muscle activation values (in contrast to optimization approaches).
Subscribe to:
Posts (Atom)